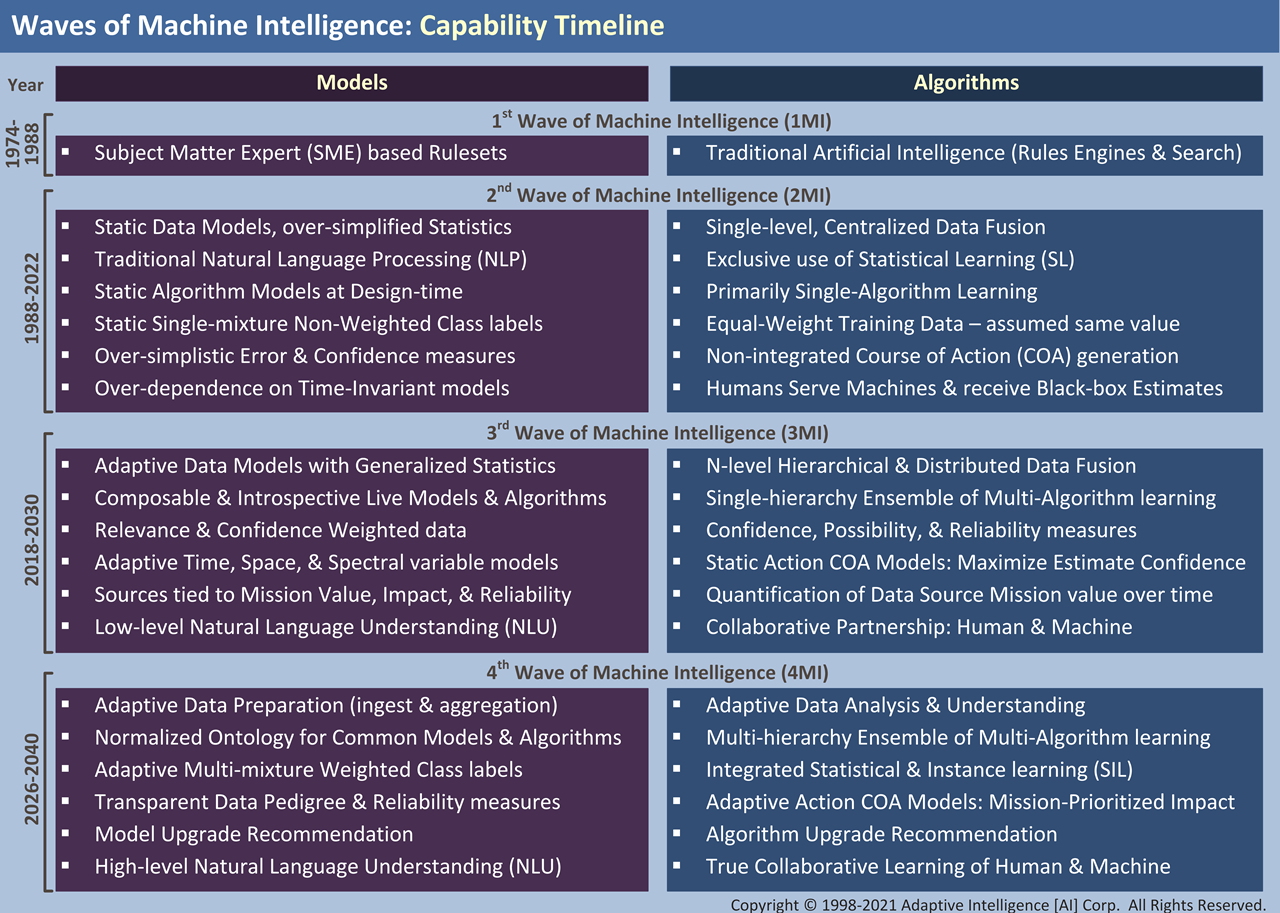
Machine Intelligence (MI) Waves
The most recent decade can be characterized by advancements in the application of machine intelligence (MI) over development of new MI algorithms & architectures. The historical first wave of machine intelligence (1MI), also known as artificial intelligence, is characterized by static subject matter expert (SME) based deterministic rules or query aggregates. The second wave of machine intelligence (2MI), characterized by static models & algorithms, is predominant in the current day today. Adaptive intelligence is a forward-looking third and fourth wave (3MI & 4MI) capability, characterized by adaptive models & algorithms. The figure above shows 24 key capabilities of 3MI & 4MI that are introspective, composable, hierarchical, high fidelity, human collaborative, explainable, and adaptable and contrasts these with previous and existing capabilities. When considering the maturation of the AI community, it is helpful to consider the key periods of innovation, which we call ‘waves’ of machine intelligence (MI). The Adaptive Tactics Sapient Human Intelligence Platform (AI-SHIP) accelerates development of 3MI & 4MI solutions.
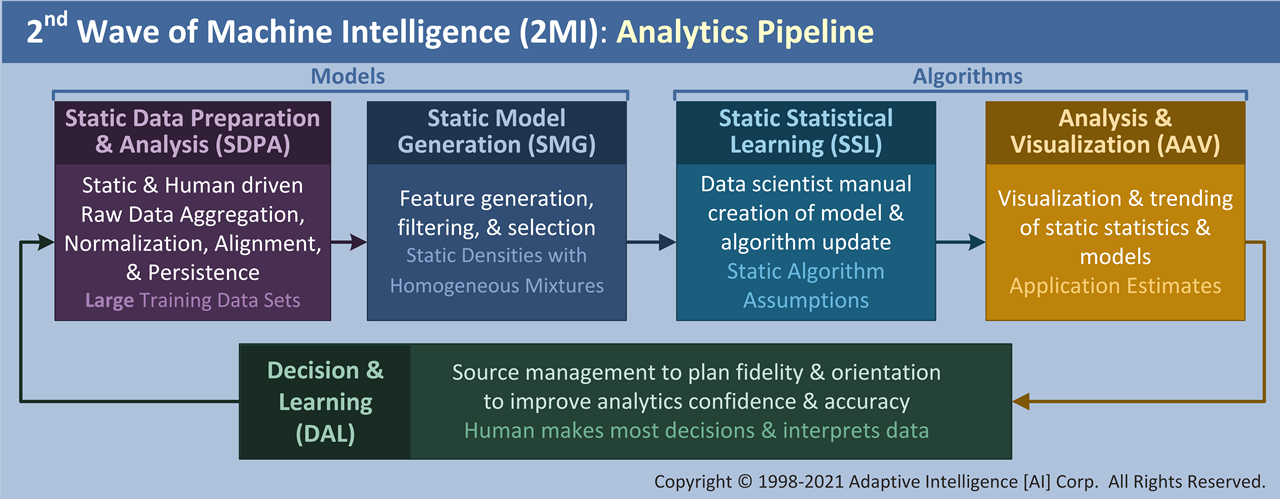
Second Wave of Machine Intelligence (2MI)
The second wave of machine intelligence (2MI) is ongoing and is primarily characterized by statistical learning (SL) methods that employ data scientist generated feature and algorithm models to estimate system state. Because the model, algorithm structure and assumptions are statically determined at design time, they become brittle over time. Changes in context caused by different tactics, techniques, and procedure (TTP) actions over time can invalidate the models. Non-optimal pattern class labels can hamstring subsequent learning.
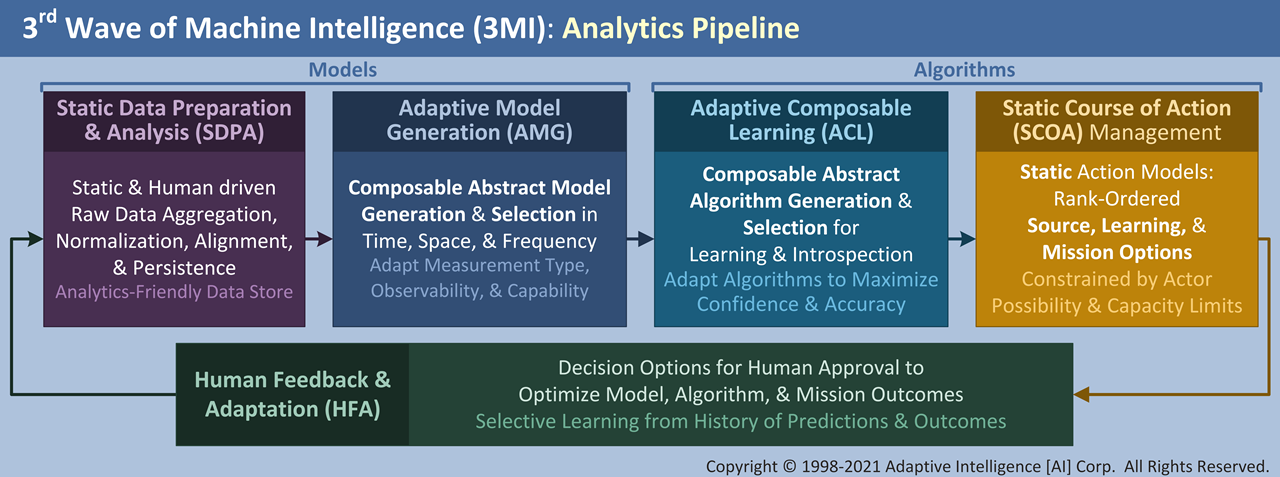
Third Wave of Machine Intelligence (3MI)
In contrast, 3MI systems are dynamically adaptive and introspective with realistic assumptions on statistics and evolution over time. The two key themes of 3MI solutions are hierarchical mission relevant activity summarization (MRAS) and live introspection of models (i.e., data statistics, distribution, and multi-class overlap in pattern space) and algorithms (i.e., machine learning algorithm configuration, accuracy, precision, recall, and Cohen’s Kappa) to adaptively adjust system configuration in response to changes in context and data statistics over time. Key capabilities of 3MI include:
- composable, robust, & realistic adaptive models with no distribution assumption;
- pattern class labels are dynamic and multi-mixture;
- statistical distributions are as non-parametric as possible; and
- the math and signal processing are generalized for distribution.
The next generation of artificial intelligence (AI) will be implemented with a 3MI approach that is introspective, adaptive, and decision oriented with human analysts as partners to generate joint impact on mission results. The figure above shows the key steps in analytics processing, where the dynamic, introspective, adaptive, and human partnership interactions are summarized.
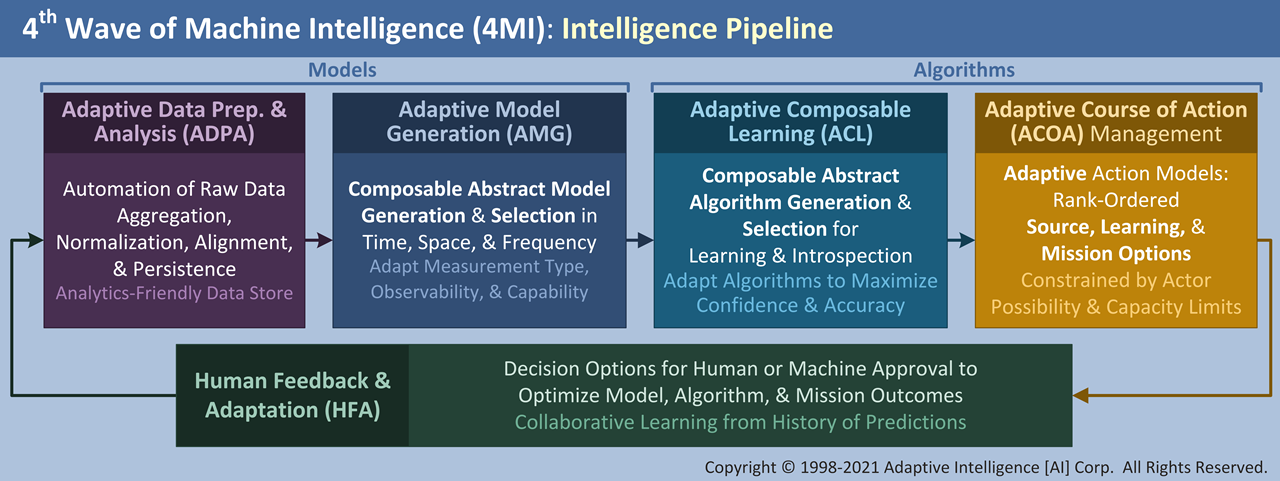
Fourth Wave of Machine Intelligence (4MI)
The intelligence pipeline in the figure above for 4MI contrast with 3MI in that data preparation and analysis becomes adaptive instead of static to automatically respond to changes in raw data formats, contents, and meaning. In addition, course of action management becomes adaptive so that action models automatically respond to contextual or concept of operations (CONOPS) changes.